3) The Knowledge Refinery
- Jay Stow
- Sep 8, 2020
- 10 min read
Updated: Sep 11, 2020
Part 3 of the 12-part series - 'A Grand Machine to Beat Covid-19' - explores how the Machine accumulates, processes and utilises data and information… systematically refining raw inputs into valuable knowledge outputs.
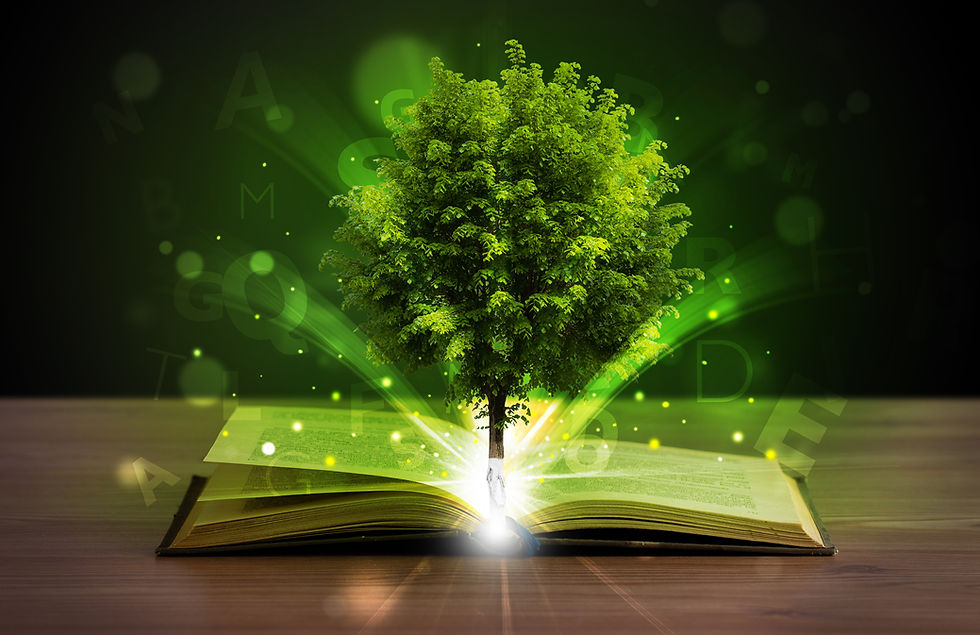
The Knowledge Refinery collects information and data from multiple sources. These inputs feed into the Machine to be systematically processed, analysed and innovated through a series of crowdsourcing mechanisms. Ultimately, the information is refined into knowledge outputs, including insights and understandings, theories and predictive models.
Information
All sorts of qualitative information needs to be collated: research papers and academic articles; organisational reports and literature reviews; policy statements and journalistic investigations; best practice guides and pandemic management advice; everything relevant to C-19 and the problems that it causes. The cogs (online volunteer workforce) are tasked with drawing together this vast library of documents on the MMM platform and arranging them into an organised database.
The crowdsourcing process is highly systematic and, where appropriate, utilises specialist crowds (cogs with relevant expertise, such as scientists or doctors). Reliability is ensured through numerous rounds of cross-checking and multiple layers of oversight. The documents are tagged with salient details (publication date, origin source, type-of-document, subject area, level of technicality, etc.), and linked to closely-related material. The cogs also write brief descriptions of the content, add search-term-tags and use rating systems to help evaluate and prioritise.
The database enables precise, sophisticated searching and the MMM’s algorithms actively try to understand what individual users are interested in, so they can be signposted to further information. The library can be visually presented as a tree, with multiple layers of division and sub-division branching out from the trunk. Detailed setting controls enable the projection of the archive’s structure to shapeshift according to personal preferences… and the user can zoom in on different areas, or study the interconnections between documents (e.g. citation and reference webs). Currently ongoing research projects are tracked and monitored, with the system facilitating early access to initial drafts and results.
The cogs can also build and maintain wiki-style resources, for example: a web-page comprehensively summarising national, state and regional social distancing and lockdown policies. The social networking aspect of the platform facilitates public discussion around key subjects, with the most interesting debates highlighted for prioritised presentation. And there’s various other useful tasks the multi-talented crowd can undertake, such as translating important research papers into different languages.
Data
The Knowledge Refinery also systematically processes quantitative information, as data feeds into the Machine from numerous sources: government statistics and organisational datasets; research data and experimental results; public transport passenger numbers and mobile-extracted geo-data; medical records and genetic maps; everything relevant to fighting the pandemic.
As an example, let’s follow the path of British C-19 infection data into and through the MMM. The UK government releases this data at the same time every day and the crowd coordinate to immediately begin feeding it into the Machine. The information is processed as it flows in – enriched with detail and made interoperable (usable) with other datasets. This involves converting the data into a universal format and tagging on salient meta-data, such as: publication date, origin source, definition of infection, environmental context, data-gathering methodology, etc.
As before, the process employs specialist and non-specialist crowds and incorporates multiple rounds of checking. AI software is used to speed things along where possible, but these technologies can have frustrating limitations (e.g. able to copy the numbers, but not always able to identify the correct boxes to put them in), so there are plenty of ‘simple’ data tasks that still need to be done by humans. The work can be quite dull, but as with most crowdsourcing processes, there’s plenty of scope for ‘gamification’.
To use an oversimplified example – copying data from one box into another can be turned into a contest, similar to the card-game ‘Snap’, with half-a-dozen online players racing to add numbered cards together to match the input figure (and put it in the right box). Rules are artfully calibrated to ensure the game is entertaining, whilst also inputting data correctly. And probably, after observing enough games, the AI software can learn to do this particular process autonomously.
As well as pumping in from every available feed, data is sourced from the MMM cogs directly. Platform-users can choose to donate their personal health information, to be used by researchers in the fight against C-19. On a basic level, a daily diary subjectively recording individual and household health should be useful in tracking the pandemic. Detailed medical records, and personal genetic data, would add even more value (although obviously, collecting and using such sensitive information entails numerous ethical and technical complications).
Systematically linking the world’s C-19 open datasets together could draw people to the MMM platform en mass, potentially enabling the construction of a personal-health database of unprecedented scale. All this concentrated information should exert a gravitational pull, encouraging organisations to open up their own datasets and feed them into the Machine. Big tech companies may donate to the Cause and specialist Coronavirus apps and projects could systematically link themselves into the platform. Hospitals, care homes and governments might choose to feed in new information directly. In return for submitting their data, these organisations would see it rapidly enriched, enhanced, made universally interoperable… and deployed in the fight against C-19.
So, the Knowledge Refinery sucks and pumps in information from multiple sources, data flowing through a maze of feeder tubes into holding-basins, to be processed by a carefully sequenced combination of crowdsourcing and AI mechanisms. And then flooding down into the great, glass tank: an information reservoir of enormous value. Where it’s ready to be superheated in the intense blaze of human ingenuity and imagination… then cooled off under the calculating rationality of scientific evaluation. Before ultimately: solidifying into concrete knowledge.
Complex Knowledge Challenges
Having accomplished the relatively simple tasks of gathering, formatting, enriching and filtering this compendium of C-19 information and data, the cogs are charged with a progression of evermore complex ‘Challenges’.
‘Data Manipulation Challenges’ call for the development of new ways of manipulating data and making diverse sets more interoperable. For example, official C-19 death rate statistics could be made more accurate by combining inputs from other mortality-related datasets. So the cogs are tasked to develop systematic processes for usefully bringing these information sources together.
‘Data Analysis Challenges’ require innovators to search the data for clues and insights, identifying significant patterns and trends. Specific questions include: What’s particular about people who only suffer mild effects from C-19? What’s special about those who get it without experiencing any symptoms? What’s distinct about individuals who suffer severely (especially those not-obviously in an at-risk group)? More open-ended questions might be as straightforward as: What interesting patterns can be observed from this data?
‘Theory Challenges’ invite the cogs to come up with theories regarding C-19 and articulate them in the form of falsifiable hypotheses. Especially interesting ideas can then be reformulated as ‘Research Challenges’, tasking innovators with proving or disproving such propositions. More general Research Challenges cover the whole spectrum of Coronavirus-relevant science. Helping to guide and structure scientific efforts and meritocratically allocating funds by rewarding top entrants with grants, to finance further research. The MMM can also facilitate peer reviews, either by replicating traditional approaches (with higher efficiency), or by enabling new, open systems.
Individuals enter these kinds of Challenges by submitting their ideas, documents or research papers for open display on the MMM platform. Others can then read and comment, engaging with the concepts and up-voting anything they find especially noteworthy. At set intervals of time (and after careful crowd-filtering) specialist judging panels assess the submissions and award them points, based on factors such as originality, usefulness, robustness, etc. Top entries are highlighted by the Machine and everything credible is added to its ever-increasing treasure-trove of information and knowledge.
Forecasting Challenges
The complexity of the tasks ramps up another notch, as the innovators of the world are invited to participate in ‘Forecasting Challenges’. For example, the ‘Infection Forecasting Challenge’ requires cogs to build models that can predict infection rates, and the geographical spread of C-19, over the next 10 days.
Participants go through the data, looking for patterns and trends, generalities and specificities, rules and exceptions. Analysing and synthesising the information… exploring theories and hypotheses about how the Coronavirus spreads and what factors are most significant in enabling or disabling the process. And there’s so many complications that need to be taken into account: How do official records relate to the actual reality on the ground? How is this affected by the testing regime? How much of the true picture is missing?
Innovators build their models and submit predictions, to be tested against the actual data as it becomes available. The most accurate estimate wins and innovators who rack up a few days of best-in-class forecasts go to the top of the leader-board. Others must improve their models and simulations to outperform the competition and leapfrog into pole-position themselves. Thus, the prediction contest carries on, as a continuous rolling programme.
Earlier models will struggle, but as the competition goes on, system dynamics encourage constant progress – favouring detailed ‘generative’ models over more simplistic statistical systems. Over time, the quantity and quality of data inputs increase, cumulative efforts to analyse and synthesise knowledge build up, new insights and theories emerge and blossom. And all the models are rigorously tested, openly and objectively – a Darwinian process that comprehensively sorts the wheat from the chaff, in the most scientific way possible.
All current predictive models should be tested through the MMM and new innovators are encouraged to come forward with novel systems. The network facilitates team-building and collaboration, actively connecting virologists, data analysts, health workers, etc. and inspiring them to make models cooperatively. Some software programmes will be developed using an open source volunteer approach, whilst others will be built by professional teams from university departments, private companies, etc. Ideally, all would show the complete workings of their models, allowing others to suggest improvements, or borrow parts of the system (in return for recognition and credit).
The MMM will systematically recognise sub-categories of success and failure. Perhaps, some models work better applied to urban areas, whilst others provide superior forecasts in rural environments. Some might be good at predicting how public transport policy impacts infection rates, whilst others better account for the effects of face-masking practices. Can these models be integrated to create a whole that is more than the sum of its parts? As the innovation ecosystem evolves, such productive cross-fertilisation should happen again and again. After a while, the best-performing models will likely be combinations of the most successful ancestor models… demonstrating the power of the approach.
As well as infection rates, similar contests will be established to forecast all sorts of things. Making predictions regarding death rates, treatment outcomes, how the disease affects different sub-populations, how immune systems evolve post-infection, etc. Disease progression modelling and simulation demands especially detailed knowledge of the Coronavirus. Every key metric or measure associated with the pandemic requires its own specially-focused Forecasting Challenge.
And then, on the highest level, there’s the ‘Grand C-19 Forecasting Challenge’, where competitors/ co-operators need to predict all of the facts and figures using an integrated system – likely an amalgamation of the most successful lower-level models.
Of course, the forecasting models won’t ever be able to predict the future exactly and will doubtless experience many failures, but the Darwinian dynamics of the competitive/ co-operative system means accuracy will continuously improve. The only scientific approach to forecasting the future, is to objectively, comprehensively and rigorously evaluate systems that have attempted to do this in the past. Therefore, the best MMM model, will (by definition) always be the best model currently in existence – the ‘Gold-Standard’.
One of the interesting aspects of the Forecasting Challenges is that they enable systematic application and testing of knowledge and theories. Consistently successful modelling algorithms will need to be based on robust insights and theories, regarding: how C-19 works, how it affects people, how it spreads through populations, etc. Thus, knowledge is refined through continuous evaluation, feedback and iterative fine-tuning of these predictive models.
Challenge-Structured Innovation
All of the MMM’s activities are structured into ‘Challenges’ – projects defined by their central purpose. Multiple Challenges connect together into holistic ‘Challenge Programmes’, whilst individual Challenges can be broken down into various Sub-Challenges. All programmes subsume within the ultimate ‘C-19 Grand Challenge’. The hierarchy can be summarised as follows:
1. Grand Challenge – broken down into multiple…
2. Challenge Programmes – broken down into multiple…
3. Challenges – broken down into multiple…
4. Sub-Challenges – broken down in various layers of Sub-Challenge, as appropriate
There are two broad types of Challenge: ‘Co-operative’ and ‘Competitive’. Co-operative Challenges tend to focus around the basic end of the crowdsourcing spectrum – simple tasks that require little cognition. For example, the ‘Data Collation’ or ‘Data Processing’ Challenge Programmes. The Co-operative approach emphasises working together, although some gamified processes utilise competitive dynamics, as well.
Complex crowdsourcing and OI tasks are framed as Competitive Challenges – where participants make submissions to be assessed through subjective expert evaluation or objective practical experimentation. How far ‘winning’ is emphasised varies according to context. For example, Research Challenges calling for scientific papers give awards to spotlight interesting entries, but generally prioritise building up a broad range of high quality submissions. On the other hand, Forecasting Challenges put a bit more emphasis on identifying the gold-standard predictive model. Competitive Challenges often offer (sometimes substantial) financial rewards to those who make the most valuable contributions to the Cause.
It should be noted that Competitive Challenges always involve lots of co-operation, as teams come together to pool resources or integrate technologies. Thus, various ‘Collaborations’ (teams/ projects) will operate within Competitive Challenges.
Summary
In summary, the Knowledge Refinery converts information into refined knowledge, by tasking the crowd with a progression of increasingly complex Challenges. The procedure runs as follows:
1. Cogs collate, gather and generate info
2. Cogs format info to enable basic interoperability
3. Cogs enrich info with additional details and salient meta-data
4. Cogs highlight most useful info and filter out extraneous inputs
5. Cogs organise open info archive for versatile presentation and exploration
6. Crowd quality control systems monitor and thoroughly cross-check info
7. Cogs build flexible toolsets to enable advanced data manipulation and interoperability
8. System assesses data manipulation toolsets – highlighting most useful and filtering extraneous
9. Cogs analyse info to identify significant patterns
10. System assesses data analysis insights – highlighting most useful and filtering extraneous
11. Cogs synthesise info into specific theories and hypotheses
12. System assesses theories – highlighting most useful and filtering extraneous
13. Cogs test theories in practice – beginning to convert info into knowledge
14. System assesses research papers – highlighting most useful and filtering extraneous
15. Cogs develop models and simulations to forecast and predict key metrics – refining the knowledge through continuous evaluation and iterative recalibration
16. System objectively tests forecasting models – rating, ranking and identifying the gold-standard
17. Cogs integrate all info, data and knowledge into a holistic C-19 forecasting system
18. System objectively tests holistic forecasting models – rating, ranking and identifying the gold-standard
19. All info, data, knowledge and innovations are fed back in, assimilated and presented on the MMM platform… the process repeats, cycles and continues
20. Cogs work continuously to improve Knowledge Refinery – developing new systems and AI features
The refined knowledge is now ready to be conveyed to the Innovation Factory, where it will be methodically manufactured into a colourful diversity of useful technologies and innovations.
Comments